Lead a normal life at home. Safely.
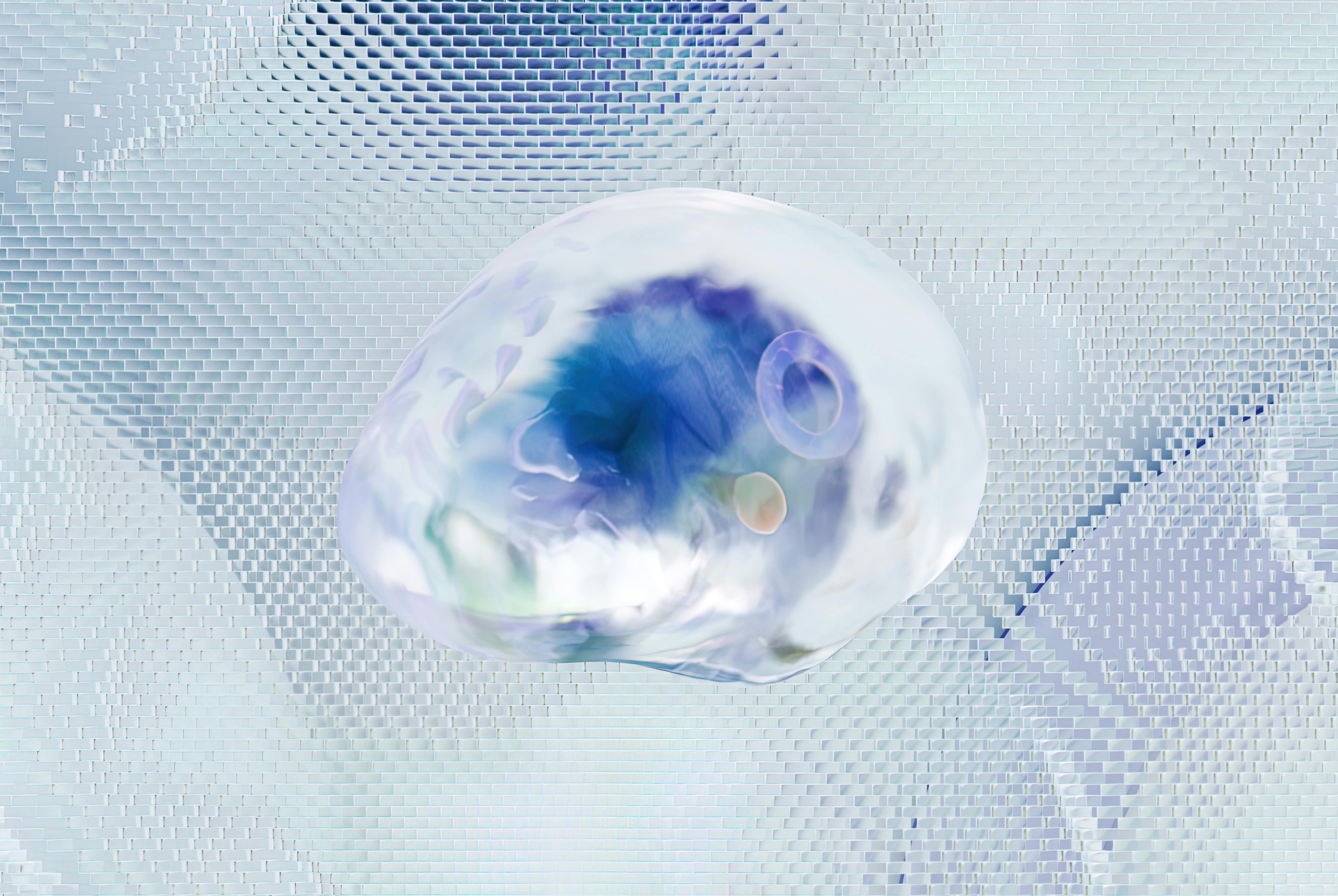
Abstract
DeepInsight™ is a novel technology designed for the ambient monitoring and assistance of cognitively impaired patients. Developed as part of the DAAN German federal 3P program—an initiative aimed at improving life for cognitively disabled individuals—it enhances daily living by enabling patients to stay longer and more safely in their own homes.
Responsibility
As inventor and Product Manager for DeepInsight™ (2017–2019), I owned all aspects of product management, including defining strategy, setting KPIs, managing costs, and conducting competitor analysis. I also led patent research, collaborated on the WIPO application, and oversaw the broader direction of DeepInsight.
I designed and built the first prototype (see solution section) to validate key concepts. As part of the larger DAAN initiative, I conducted UX research, ensuring insights informed both DeepInsight™ and other projects across the program.
Outcomes and impact
- Patent Pending
- Achieved significant visibility and recognition.
- Secured multiple strategic partnerships and follow-up projects with companies in the medical sector.
Problem
In developed countries, the population above 65 years of age is rapidly increasing, and so are cognitive degenerative diseases such as Alzheimer’s dementia. By 2025, the caregiving system will reach its maximum capacity. There is an urgent need for the decentralisation of our current health system.
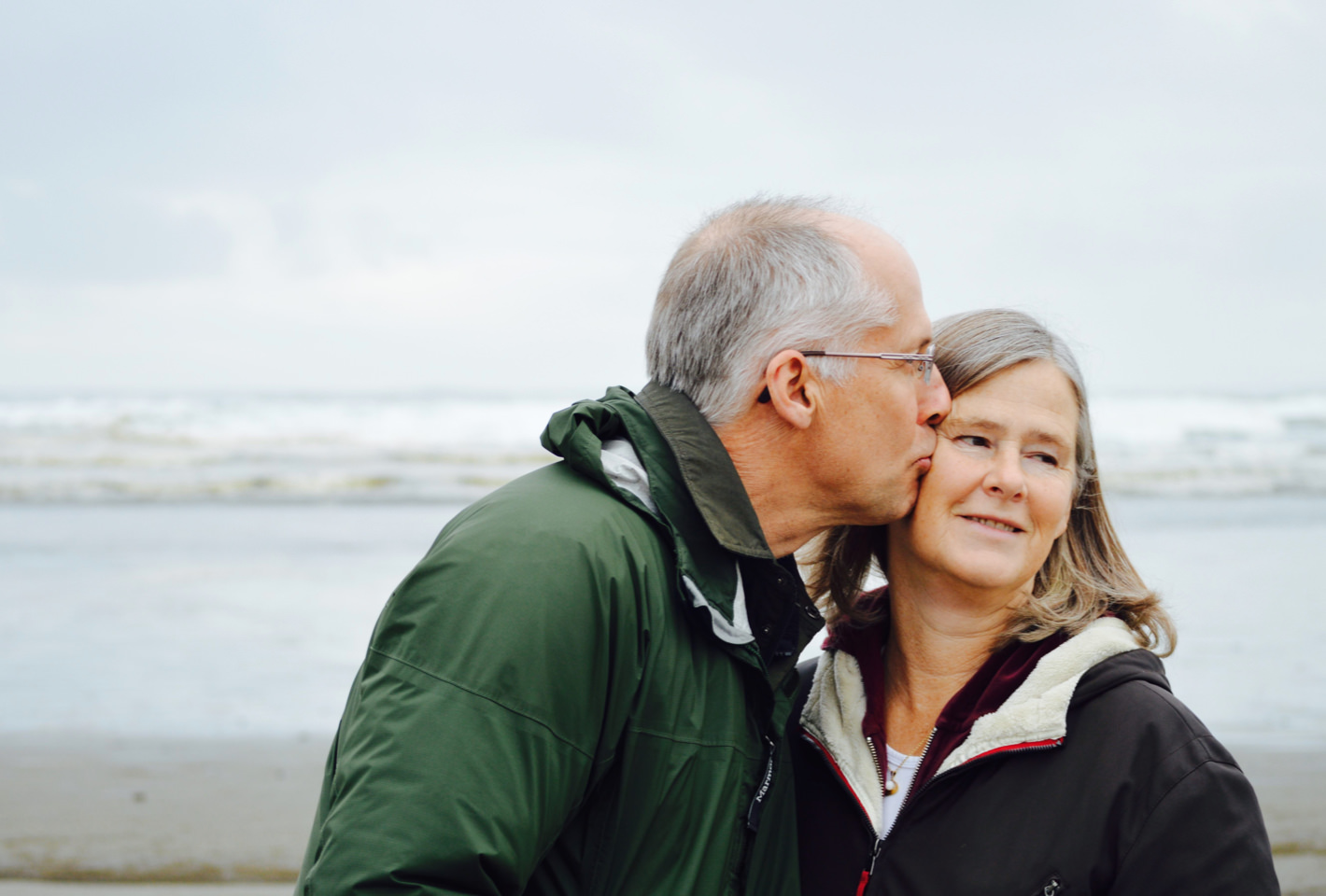
Here are a few key numbers to help understand the problem at hand:
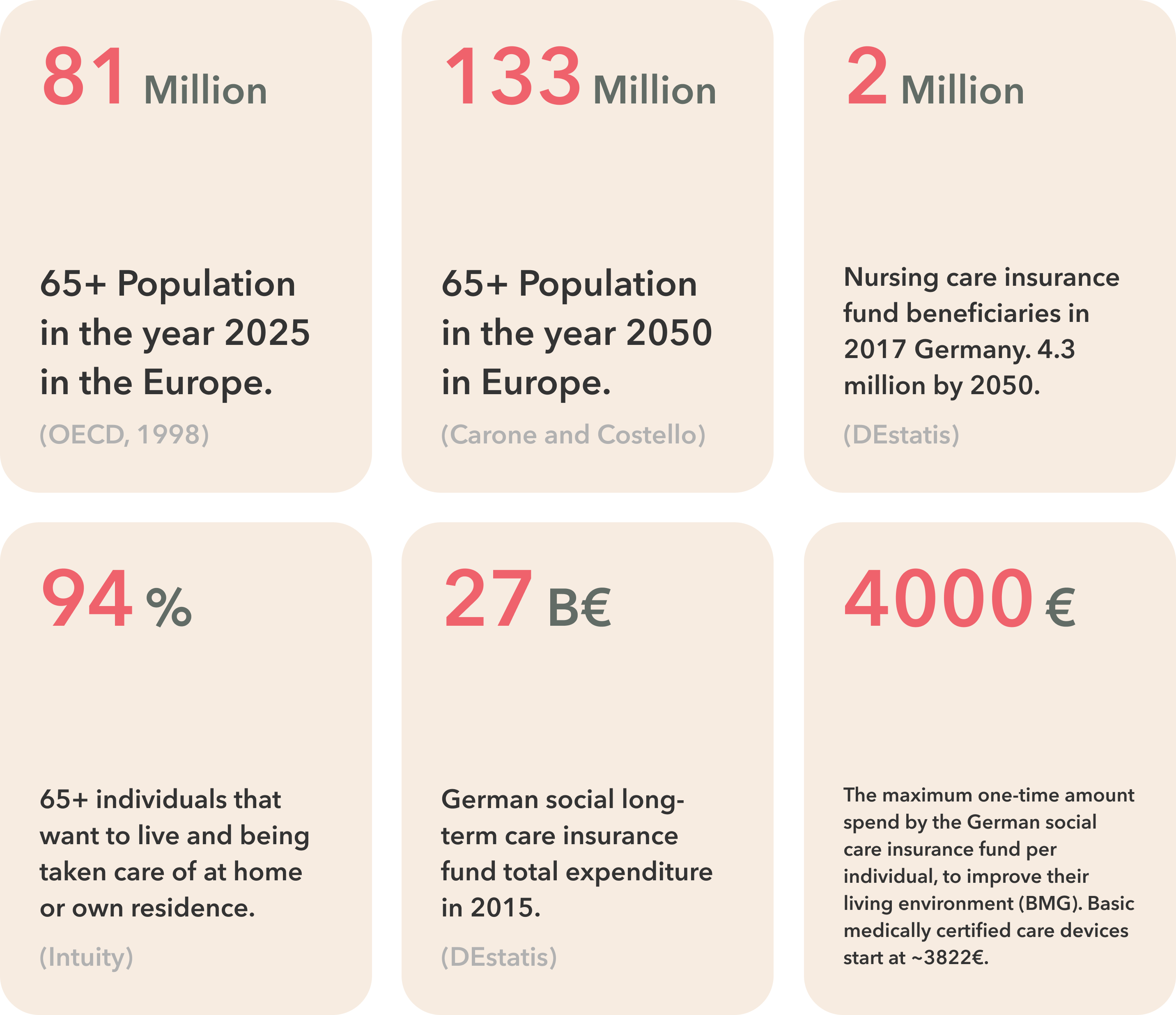
Project backround
DeepInsight is the third and final product of the DAAN (Design Adaptive Ambient Notification) German federal 3P program, which spanned multiple years. DAAN aimed to allow public entities (such as universities and clinics) and private companies to work together on projects and technologies that would enable cognitively disabled individuals to lead better and safer lives. As a media lab, we were heavily involved in developing new ideas and prototypes, working closely with medical professionals and universities.
Our contributions were:
I was responsible for Caloo and DeepInsight. I also served as the research lead for the DAAN project, conducting numerous studies that shaped our products and informed both internal and partner product strategies in healthcare, dementia, caregiving, and geriatrics.
Design process
Having worked for multiple years in R&D and Product design during Caloo and Kopernikus, I had the privilege to continuously collaborate with multiple stakeholders and experts in medicine, engineering, behavioural science, psychology, and research.
It was a humbling and unique experience. But it wasn’t without its flaws. I noticed the following issues, which could partially be attributed to the 3P nature of the project.
My key learnings occurred in the following areas:
I concluded that to create a truly usable, valuable, and enjoyable product, one would have to start with the user experience and work backwards to the technology. Thus, the first step towards the development of DeepInsight was made.
Strategy and Design Methodology
I took the role of a product lead (manager) and started the process of developing a novel system. However, there was a challenge that was set by the federal program (DAAN): I had to come up with technology in any shape or form. Balancing design research while scouting for new technologies, not knowing if and how they could be implemented, was truly challenging and unique.
I adjusted the double diamond to fit the project’s needs.
First, I allocated the UX research section of the process to myself. I already conduct multiple UX research studies. The main goal in this project would be to correctly synthesise the results, extract the most informative insights, and define new KPIs.
Second, I replaced traditional design ideation methods with an engineering technique called the theory of the resolution of invention-related tasks (TRIZ), or the theory of inventive problem solving (TIPS). TIPS follows an algorithmic approach to the invention of new systems. Its core idea starts with the concept of a “perfect product” and then, using 40 principles of technical evaluation, derives a reality-based solution. More on that later.
Defining the Problem and KPIs
After synthesising my research and liaising with medical experts, I came up with the following problem statement for the DeepInsight project:
“How might we build an ambient system that could assist and monitor dementia patients at home, without them having to interact with anything?”
I then defined the following KPIs and metrics:
Competitor Analysis
I listed a few smart devices and created guidelines on how to measure stimuli levels qualitatively. After giving my colleagues a short seminar, I asked them to evaluate them based on those guidelines.
Analysing the metrics using traditional UX methods such as by 2×2 matrices would not suffice. The dimensionality did not cover all the stimuli. To overcome this, I created a 3D data visualisation. This turned out to be too complex to visualise on keynotes, and a simple spider chart did the trick. Each corner was coded with one sense. The webs represented the stimulation level. The findings were then stored in an Excel table.
To make everything more interactive and to better visualise the results, I transferred the data to Plotly.
An example can be seen below:
Example. Smart Speaker Evaluation
Evaluation for traditional and non-traditional senses
Ideation
Using the TIPS methodology, I came up with multiple “perfect product” statements.
These statements are technical contradictions. The process dictates that closely overcoming these technical contradictions using the 40 principles of technical evaluation and extensive patent/literature review would directly result in novel inventions.
A perfect system should:
- Know and analyse everything, but be invisible to avoid cognitive overload.
- Be highly effective and yet free.
- Assist the user without communicating with anything or anybody.
- Be one device that can manipulate or stimulate all metrics (see chapter on metrics).
- Be secure and yet easy to maintain and access.
- Be a high-end product yet highly scalable.
After TIPS practice rounds with the team, it was time to start ideating. We listed the principles that needed to be used and proceeded to the research phase.
Here you can see how I organised this phase:
Invention
Multiple ideation sprints were made, yet we could not solve the scalability problem. It was quite challenging to find a solution or technology which would allow DeepInsight to scale fast.
Then one night, I had a revelation. I remembered a technology called nonintrusive load monitoring (NILM). Simply put, it gives insight into a household’s power usage to a degree where one can classify the watched TV shows.
NILM works in conjunction with modern smart meters. The good news is that Germany plans on making smart meters obligatory in every household by 2025. This solves the scalability issue.
One problem was that NILM cannot analyse water usage, which is essential if we want to know someone’s urine control, bathing, and showering activities. To overcome the issue, I came up with the following idea:
Pair a modified NILM smart meter with a fully digital radar sensor smart water meter (SWM). The SWM is easily mountable on any central water pipe and can monitor the water consumption in the millilitre range. The sensors’ (power + water) data output would then be processed using a novel AI to classify home activities.
To better classify activities, I conceptualised a multi-layer AI architecture (which later became part of my patent).
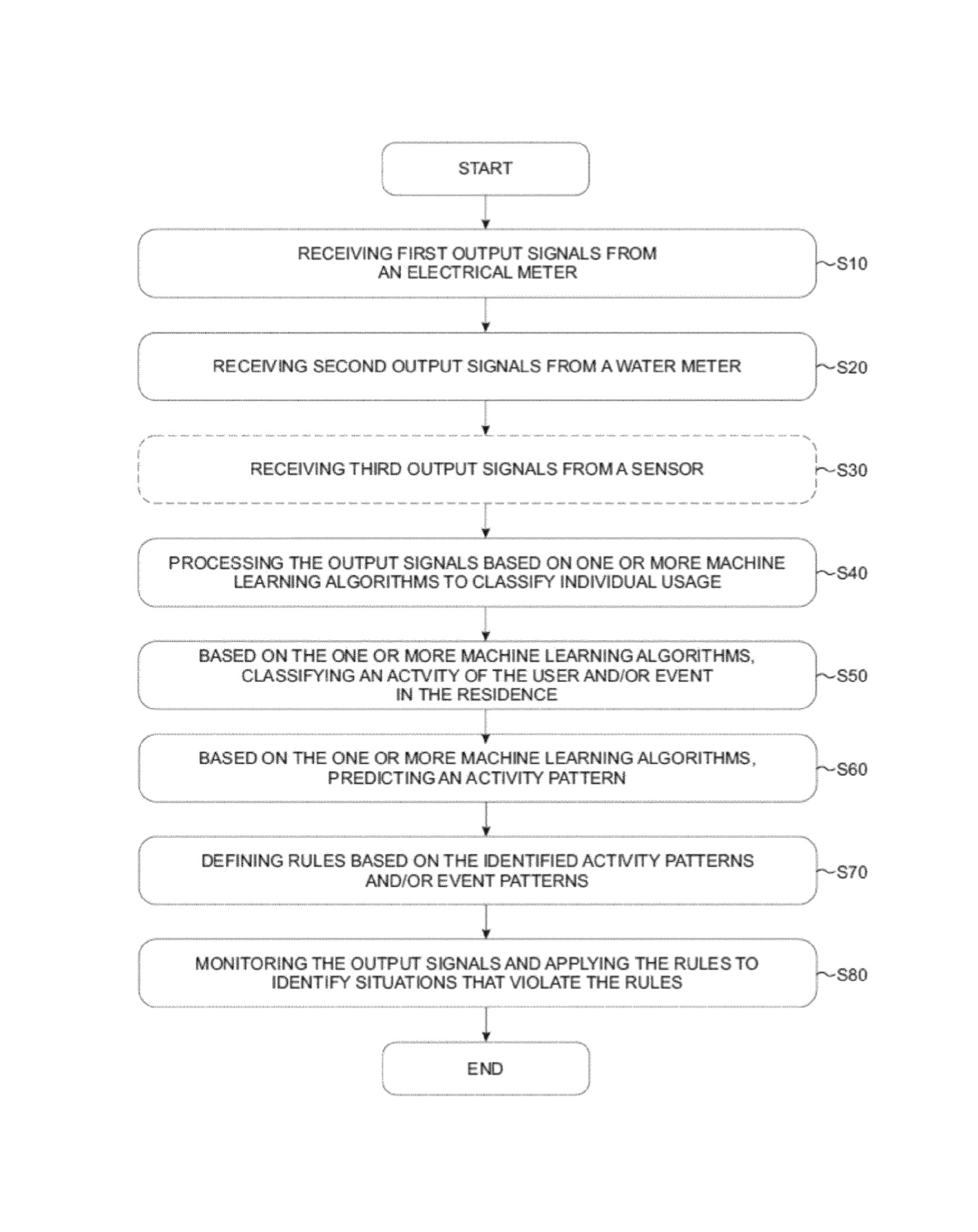
I liaised with an Machine Learning experts who very much liked the idea of separating each classification step, and I proposed a classification structure. Below, you can find the advantages of a multi-layer approach:
Early Prototypes
The initial functional prototype of the system was designed with simplicity in mind. A timeline on the interface displayed power consumption segmented into three categories: high power usage (above 850W), mid-power usage (between 850W and 120W), and low power usage (below 120W). Colored bars represented these power consumption levels over time. The opacity of the bars indicated the predicted/expected power usage, derived from daily patterns analyzed through machine learning algorithms.
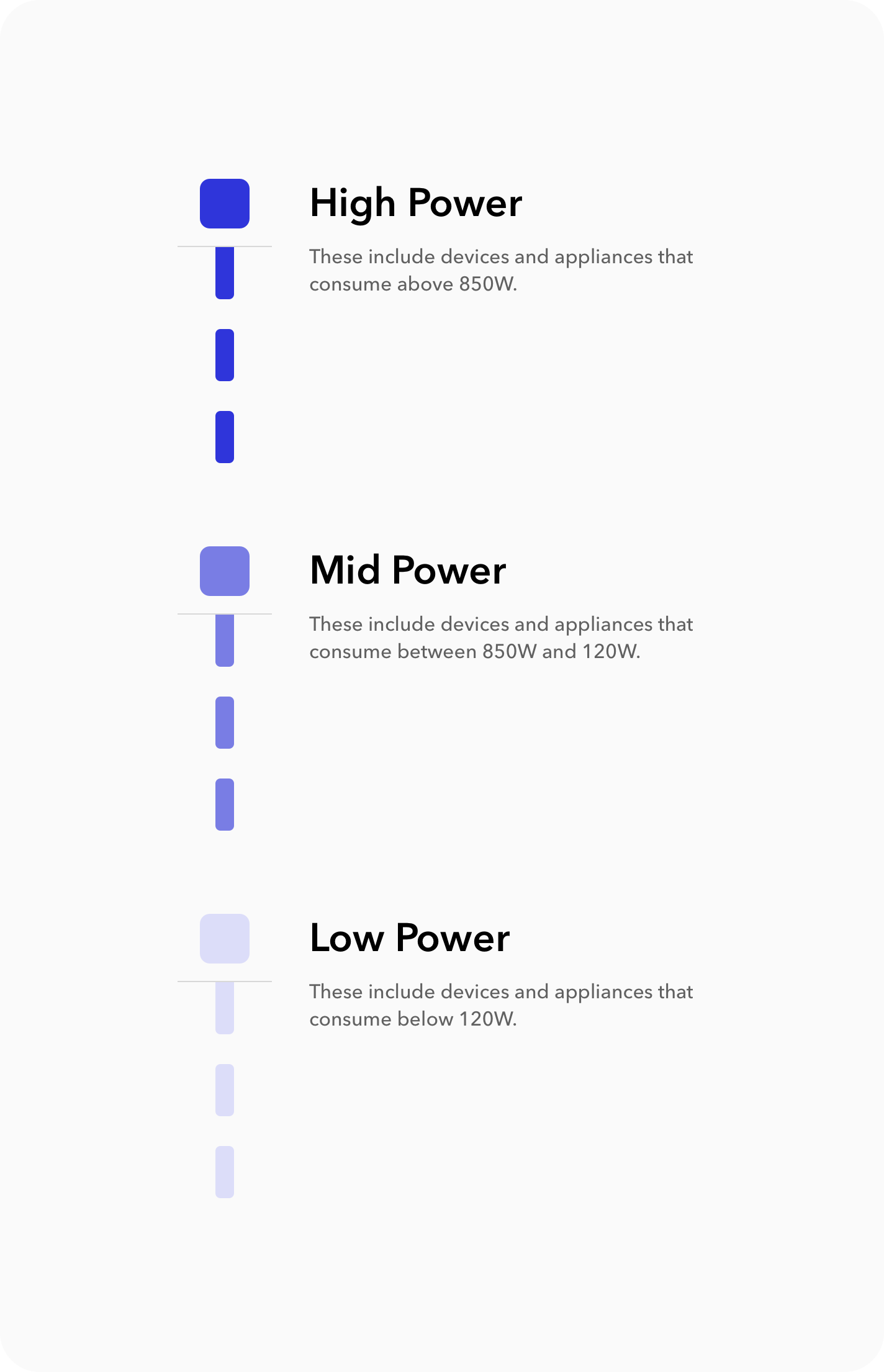
These power usage patterns served as a foundation for predicting activities of living (ADLs), such as “cooking” The system’s machine learning model classified ongoing and expected ADLs based on these patterns, offering insights into a patient’s typical routine.
When navigating into the ADL details, a caregiver or family member could review the classification accuracy of the machine learning predictions. The system presented a ranked list of the most likely to least likely activities, enabling users to better understand the patient’s behavior. Users can also manually label different activties for fruther training.
Through a connected app, family members or next of kin could monitor the patient’s current activities in real time. They would also receive alerts if deviations from the recommended ADLs occurred. Significant deviations—potential indicators of health or safety risks—triggered status changes. In critical cases, the system would send notifications to app users or emergency services, ensuring timely intervention if the patient required assistance or an urgent check-in at home.
The first prototype underwent user experience research (UXR) testing, where it was well-received and universally understood by participants. Testers appreciated the clarity and simplicity of the interface, which ensured that even non-technical users could easily interpret the data and make informed decisions.
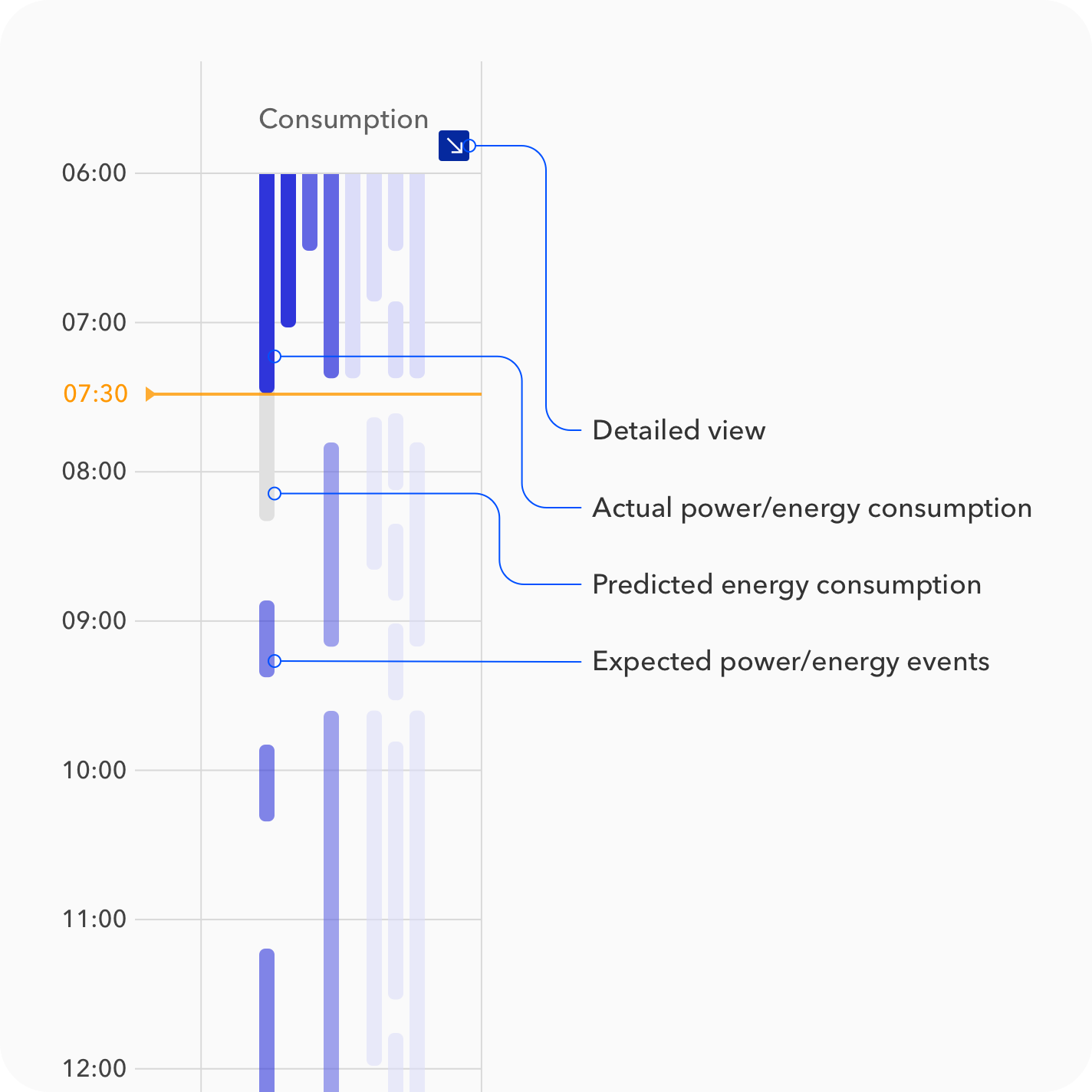
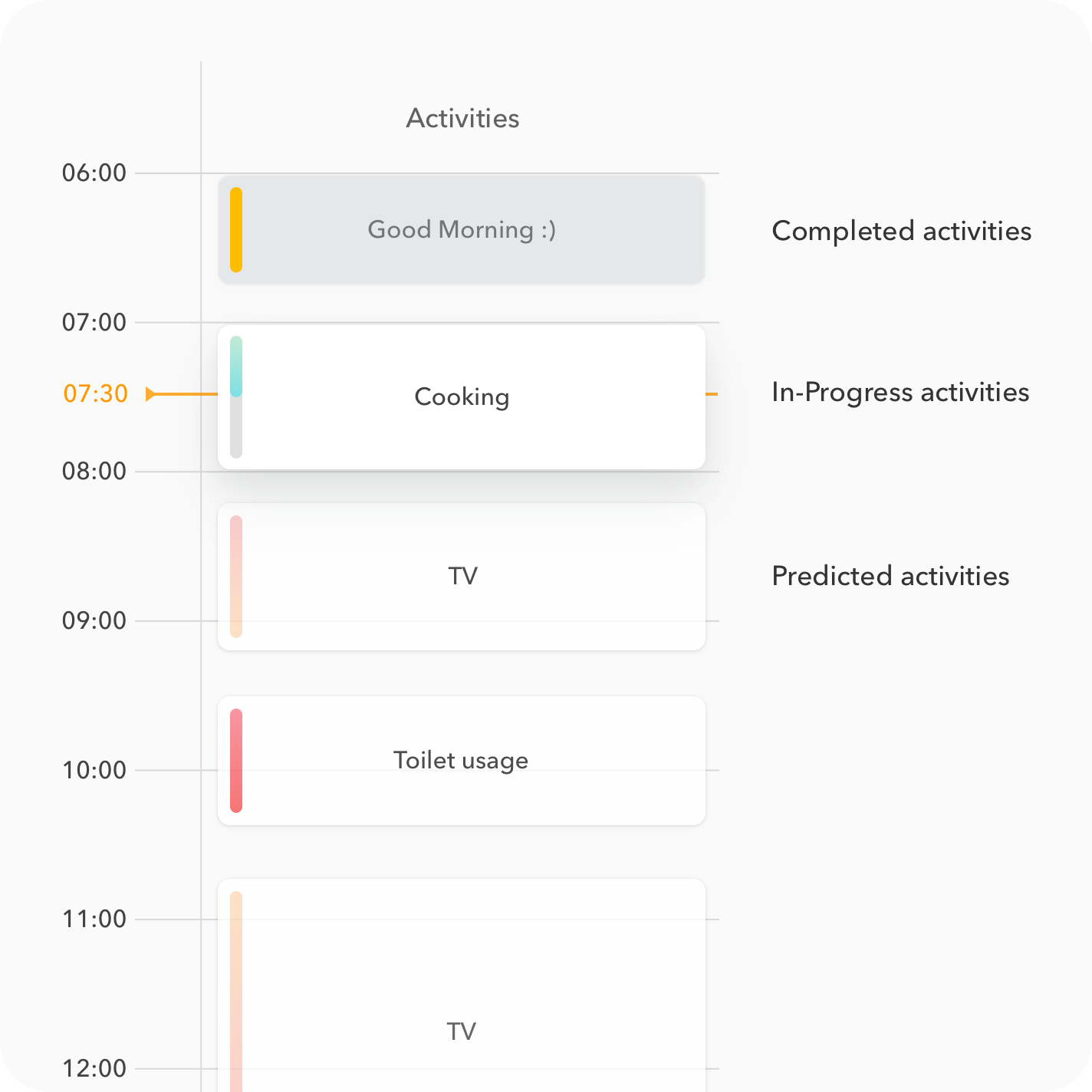
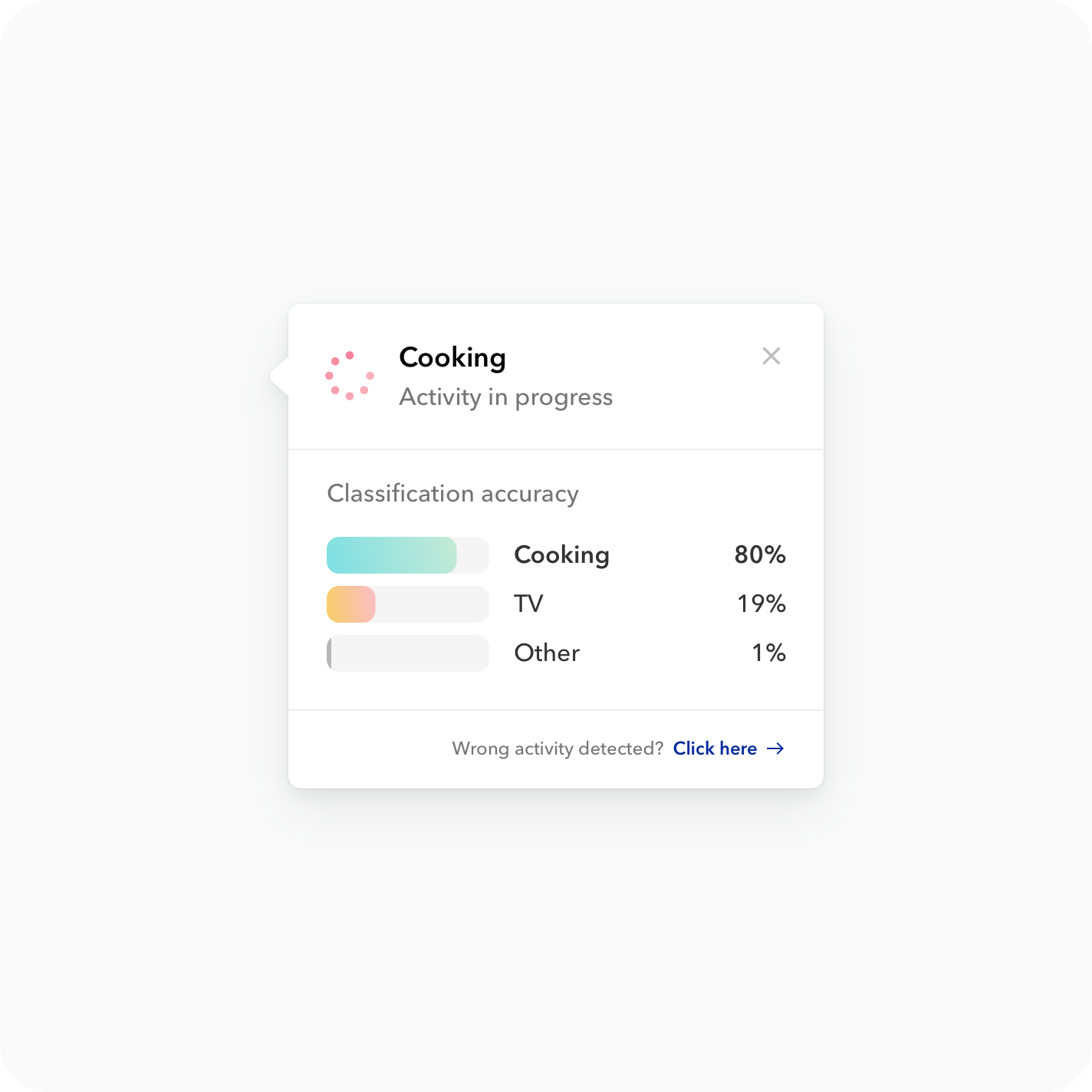
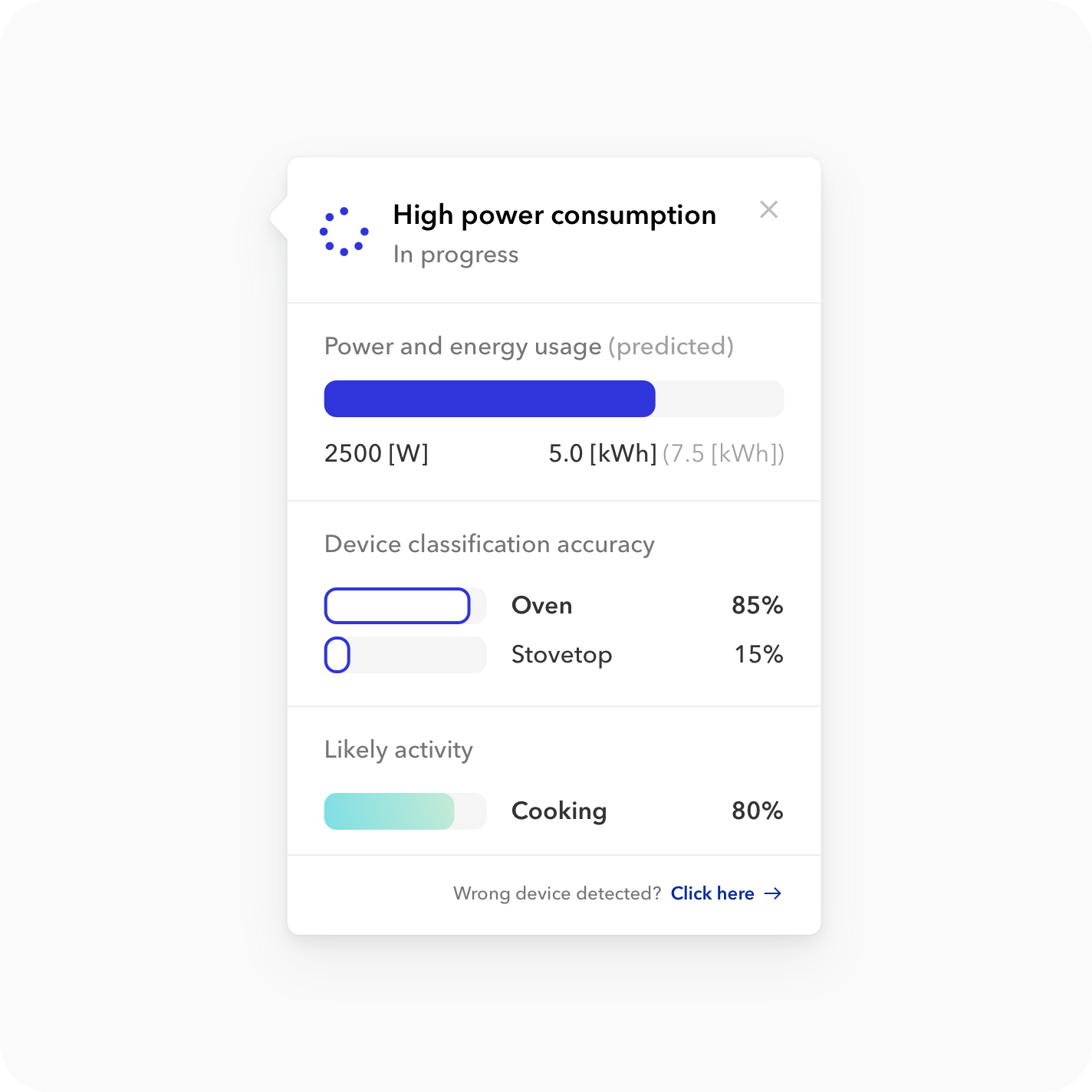
Reflection and learnings
Inventing systems with user-centered design and engineering principles is a process of constant learning and refinement. Each iteration brings new challenges, along with new opportunities to improve. Patenting the technology was another step in this journey, providing an eye-opening experience that deepened my understanding of how to protect and position innovation.
Through DeepInsight and other initiatives, we addressed critical gaps in today’s dementia technologies while also exploring bold, forward-thinking questions: How will people interact with systems they cannot see? What does a user experience look like when there is no visible interface? Can micro-radar technology help capture more precise movement data?
Though these questions were ultimately tackled in a seperate effort, the journey revealed far more than technological challenges. The projects and initiatives opened my eyes to the profound impact dementia has on families, physicians, and the healthcare system as a whole. It was a truly humbling experience.
Even though DeepInsight was a prototype, it generated significant secondary impacts. The visibility it created enabled our company to undertake many follow-up projects in the medical space, and it also established a strong reputation for us as a team committed to innovation and user-centered solutions.
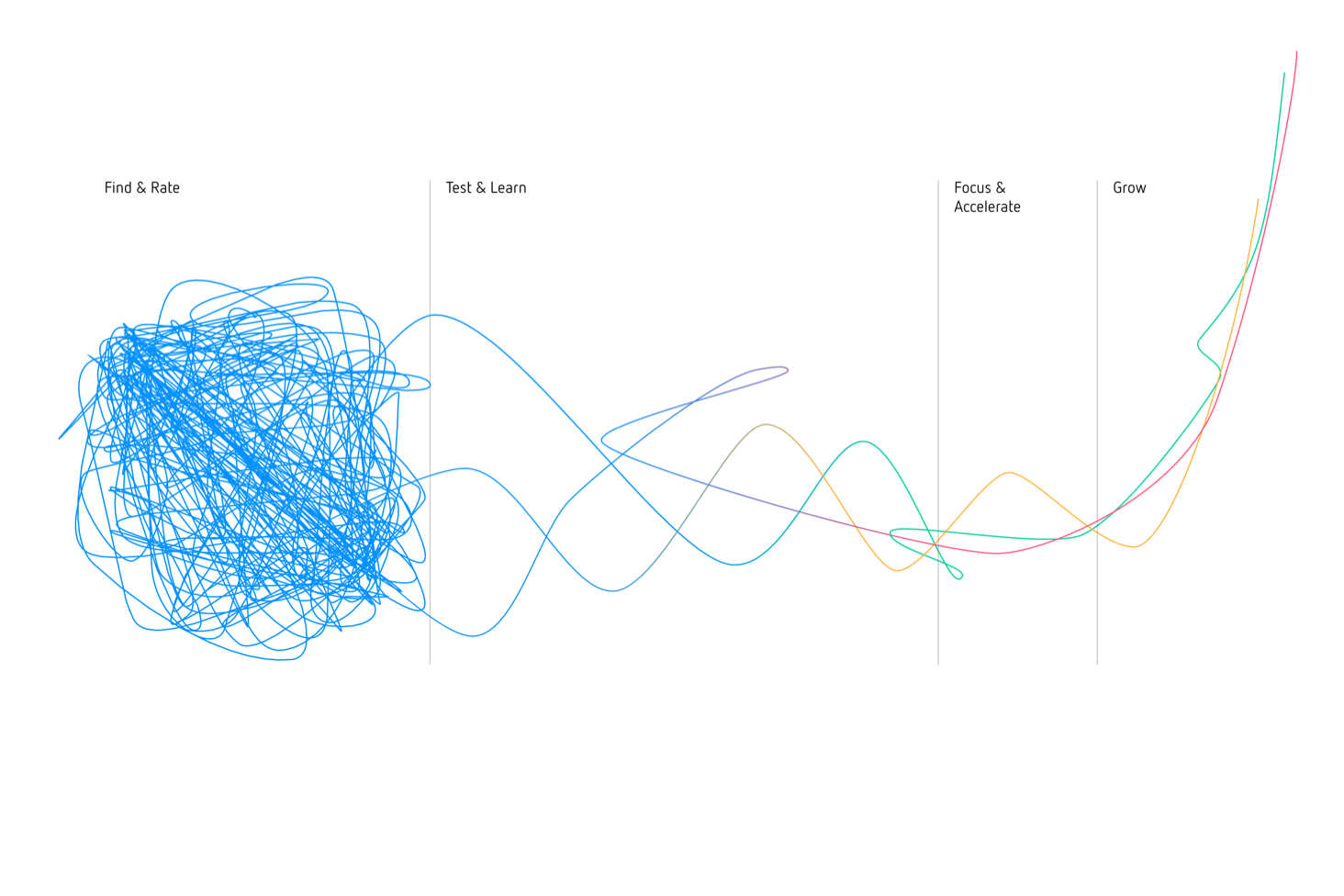